Machine Learning Models Don’t Work

Machine learning (ML) is a branch of artificial intelligence that uses data and algorithms to imitate human behaviour (Brown, 2021). It is used in a variety of financial applications such as fraud detection, automatic trading, robo-advisors, loan underwriting, and targeted advertising. Machine learning revolutionizes how we invest, trade, advertise, and do business more broadly.
Machine learning also transforms how we conduct research and generate business insights. It offers unprecedented opportunities to use big data to identify patterns and extend our understanding of mechanisms. For example, creditors increasingly use ESG information to assess default risks. Currently, this assessment is predominantly of qualitative nature meaning that the analyst screens available material and incorporates the resulting impression into their assessment. Research involving machine learning could allow us to systematize the interrelations and generate tangible and actionable insights, including quantitative prediction of credit default probability.
A key question that arises upon this new opportunity is how to integrate machine learning in research design. Is it an add-on? Or a replacement? Or does using machine learning in research require a completely new way of designing studies altogether? This article discusses different ways to integrate machine learning into research design and their implication for knowledge generation and product creation. We use ESG and credit default as an illustrative case study. Specifically, we demonstrate the applicability of an ML-driven research design approach to determine the inter-relationships between ESG factors and credit default probability.
Following the Traditional Path
Most textbooks on research design present a fairly linear structure (Bergman, 2008; Flick, 2004; Babbie & Mouton, 2001; Bryman, 2008; Bouma & Atkinson, 1995; Diekmann, 2001). Research design is understood as a set of consecutive steps that build up on one another. The graph overleaf shows an illustrative example. Researchers start by studying existing theory on ESG and credit. From this study, a research question and/or a set of hypotheses arise such as “Is there a relationship between a company’s ESG performance and credit default?” or “Companies with good governance are more likely to repay their loans.” Researchers then collect ESG and credit rating data to answer this question or test the hypotheses. Next, they analyze the data and write up the results. Voilà the research is finished!
Figure 1. Traditional approach to research design
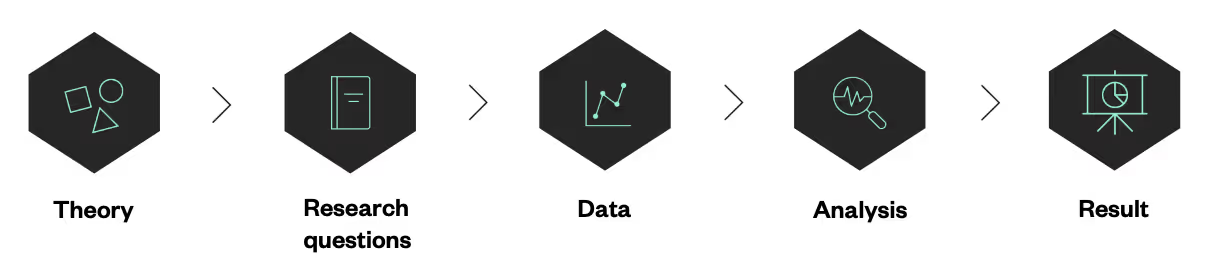
Granted, it is not quite that easy and there are some inherent flaws in this structure that we will turn to later (see also Bergman, 2008). However, let’s assume, for now, that this simple understanding is how research actually works and focus on adding machine learning to the mix.
Integrating Machine Learning the Wrong Way
Integration of machine learning in research design is based on our assumptions of what it can (and cannot) deliver. Unfortunately, sometimes, these assumptions are wrong and this can negatively affect our research design.
From a research design perspective, two simple misconceptions can provide a particular challenge. First, machine learning is often understood as a technique that can find patterns in data all by itself. Basically, machine learning is thought to take in all available data and then sort it in meaningful ways and spit out truths or even theories--an exaggerated manifestation of this idea is the movie Transcendence. A weaker but related notion is that machine learning is able to find the relevant variables by itself (Caldwell, 2018; Findlow, 2020). In the case of ESG and credit default, this would mean that we feed into the model all types of ESG indicators and a variety of credit default indicators and it will tell us which indicators are the most important and how the former determine the latter.
Second, machine learning and statistics are regarded as alternative pathways. Statistics is often seen as the go-to strategy for making inferences and testing hypotheses (Bzdok, Altman, & Krzywinski, 2018). Statistics helps us understand how a system works (ibid). In contrast, machine learning is used for making forward-looking predictions (ibid). Machine learning models help us to make decisions without having to understand the underlying mechanisms (ibid). Hence, it is often inferred that you need either statistics or machine learning, depending on your end goal. If you want to understand whether there is a relation between ESG and credit rating, you use statistics. If you want to predict credit rating using ESG, you use machine learning.
These two misconceptions lead to the conclusion that research designs involving machine learning are significantly different from traditional approaches. In particular, the assumption that we can skim or skip research questions, hypotheses, and statistics. The resulting design might look something like this:
Figure 2. Research design resulting from misconceptions of machine learning

Let’s revisit the misconceptions. It is true that machine learning is able to identify patterns in data. But these patterns are not necessarily meaningful or hold true across specific data sets. In a supervised setting and given a specific dataset, machine learning may, for example, find no relation between sports clubs and debt. It might therefore wrongly conclude that sports clubs generally don’t have debts (adopted from: causaLens, 2021; Beery, Van Horn, & Perona, 2018). Similarly, machine learning may be able to identify relevant variables, but it can also fail. Sector or organization type might not be the best metric to predict debt. More importantly, the patterns depend on the input data provided and as such are dependent on the (biased) human designing the model. In the above example, researchers might not have provided training data which includes indebted sports clubs.
"Statistics and machine learning do not represent separate paths. For most projects, both inference and prediction are important."
It is also true that statistics tends to focus on inference and machine learning tends to focus on prediction (the difference between statistics and machine learning is fuzzy and subject to much debate; Stewart, 2019; Bartlett, 2019). However, they do not represent separate paths. For most research projects, both inference and prediction are important. We want to understand the relation between ESG and credit default in order to predict credit default risk and recommend precautionary actions. Similarly, good prediction depends on some understanding of actual or potential underlying mechanisms and relevant factors. If a machine learning model is given the colors of corporate logos and first names of the last joining employee, it will likely be incapable of making accurate predictions of credit default – it will nevertheless attempt to do so. Therefore, domain expertise is as important as technical skills are.
If machine learning is not able to find relevant features or patterns by itself and if understanding the system is important but theory, research questions, hypotheses, and statistics are taken out of the equation, then research design runs two risks:
Needle in the haystack: With no theory, hypotheses, or questions to guide the inquiry, one is likely to find one-self with a needle in the haystack problem. One might run dozens of models on hundreds of ESG and credit default variables in the hope of getting a model that works and provides acceptable predictions.
Meaningful models: If one finds such a model, one might find it difficult to evaluate. Without guiding theory or hypotheses, feature importance might be hard to understand and cross-check. Do organization types or CO2 emissions reflect meaningful predictors? Will these predictors hold across context and data sets?
Integrating machine learning a better way
Let’s not get confused by misconceptions. Theory, research question, and hypotheses are important components and (often) good starting points. Since statistics and machine learning are not alternative paths but rather complementary, we may think of them as consecutive steps in the traditional research design logic. We start by exploring our hypotheses on relations using statistical methods. The thus gained knowledge is then used to design meaningful machine learning models (for a good overview of machine learning design, see Leong, 2019). This yields something like this:
Figure 3. Machine learning in the traditional design (academic setting)

Or, in a more applied setting:
Figure 4. Machine learning in the traditional design (applied setting)

Taking an alternative route
To everyone who has ever conducted research, the linear design approach may seem like a stark abstraction and simplification. Research is rarely a linear process with consecutive well-defined steps. Rather it is an iterative process with many loops of revisiting and adjusting earlier steps, sometimes even throwing out initial ideas and going back to the drawing board (for a detailed discussion see Bergman, 2008; Bergman, 2011). For example, researchers may start with an interesting question about ESG and loan repayment, but then realize that there is no data on loan repayment readily available or that they lack the necessary methodological skills. They may then alter the research question to better fit available data or skills. Researchers may also not always start with theory. They may acquire access to really interesting credit and ESG data and phrase a research question or use case that exploits this opportunity. This more fluid design is captured in Bergman’s (2008) conceptualization shown in the diagram below. The diagram emphasizes the back-and-forth between research question, data, and analysis. It allows for flexible starting points and depicts the research results as the outcome of interrelated decisions on research question, analysis, and data (Bergman, 2008).
Figure 5. Bergman's (2008, p.587) alternative view on research design
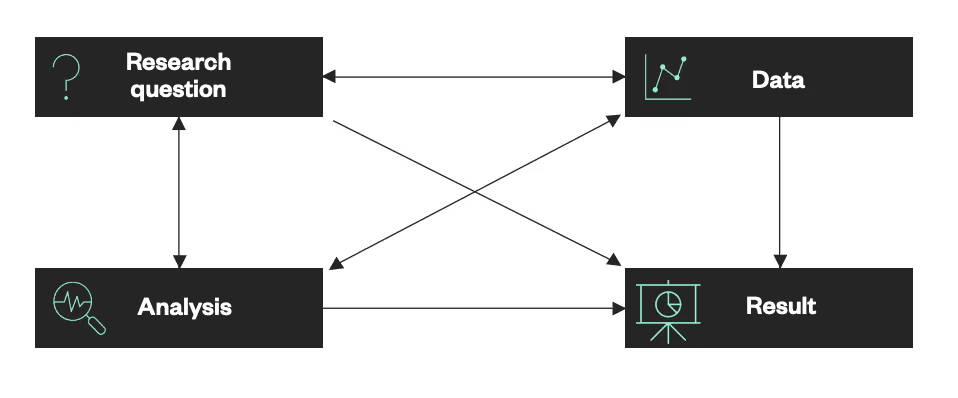
Integrating machine learning in an even better way
Let’s add machine learning by using this more flexible approach. Based on the insight that machine learning benefits from the results of statistical analyses, we may conceptualize research involving machine learning as two interconnected parts. The first part focuses on understanding the system and identifying key factors and relations--or, in an applied setting, testing experience based hunches on what could be causing the problem. This is done using exploratory and inferential statistical techniques. The second part employs the results of the first part to make predictions. Using the example of ESG and credit default, we could first understand how different ESG factors influence a company’s ability to repay its debts. Next, we use these insights to train a machine learning model to predict credit default based on a company’s ESG performance. The diagram below provides an illustration of this design.
Figure 6. Machine learning in the flexible design
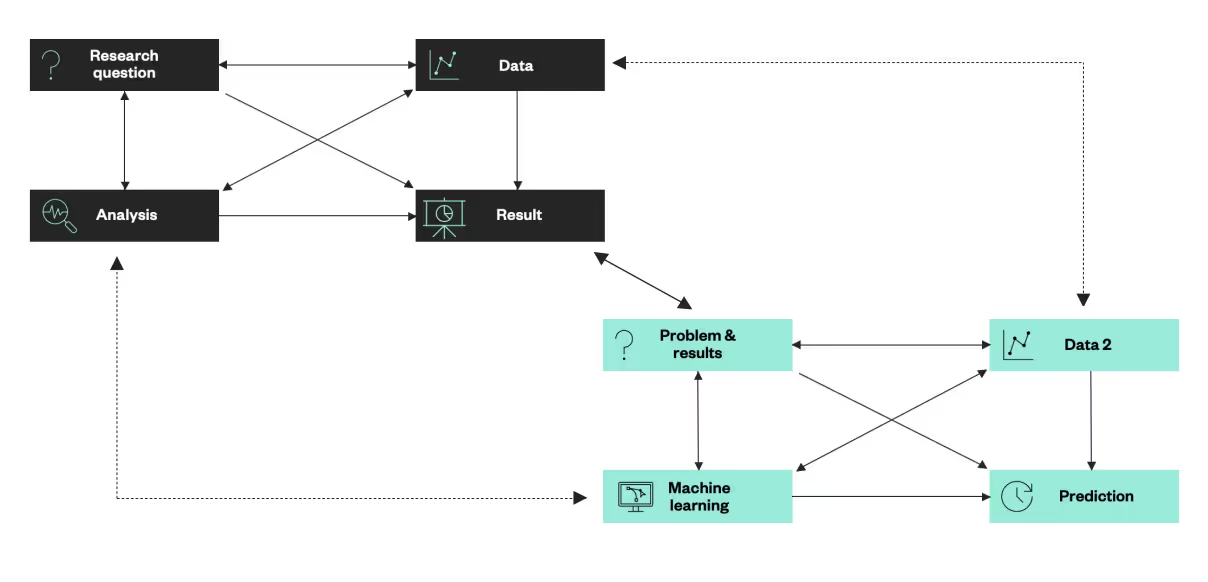
The two parts are interdependent. This interdependence goes beyond the second part building up on the findings of the first part. It also encompasses a selection of data, analysis, and problem definition. For example, the data used for the statistical analyses and the data used to train and evaluate the model should be distinct and hence we need to carefully plan data use across the different stages. Similarly, classification and regression models differ in the types of insights that they benefit from most, and this needs to be considered when conducting statistical analyses.
The two parts are also not strictly consecutive. While it is advisable to first generate a better understanding of the mechanisms and system before training a model, in later stages, back-and-forth loops are possible (in some cases even likely). For example, a model guided by the statistical analyses of the first part might not predict credit default as intended and researchers might decide to go back and conduct some more analyses to enhance the model. Or, a trained model may spark some ideas about additional relations between ESG and credit default that researchers decide to study in more detail. Or, to go even further, the outputs of a trained model such as classifications of companies can be used to conduct additional analyses leading to a quite nested structure. In some cases, researchers may even start with machine learning. This is, for example, the case when we tag data in natural language processing or interpolate or augment data. The thus tagged, interpolated, or augmented data is then used to conduct (further) statistical analyses.
Conclusion
Machine learning offers unprecedented opportunities to change not only how we invest and do business, but also how we conduct research. However, machine learning is not magic! It can’t do all the thinking for us (just yet). To benefit the most from a machine learning model and generate actionable insights that financial institutions, investors, and corporates can rely on, we need a solid research design process, wherein examination is guided by experiences or theory, and statistics and data science go in tandem.
Read More Research
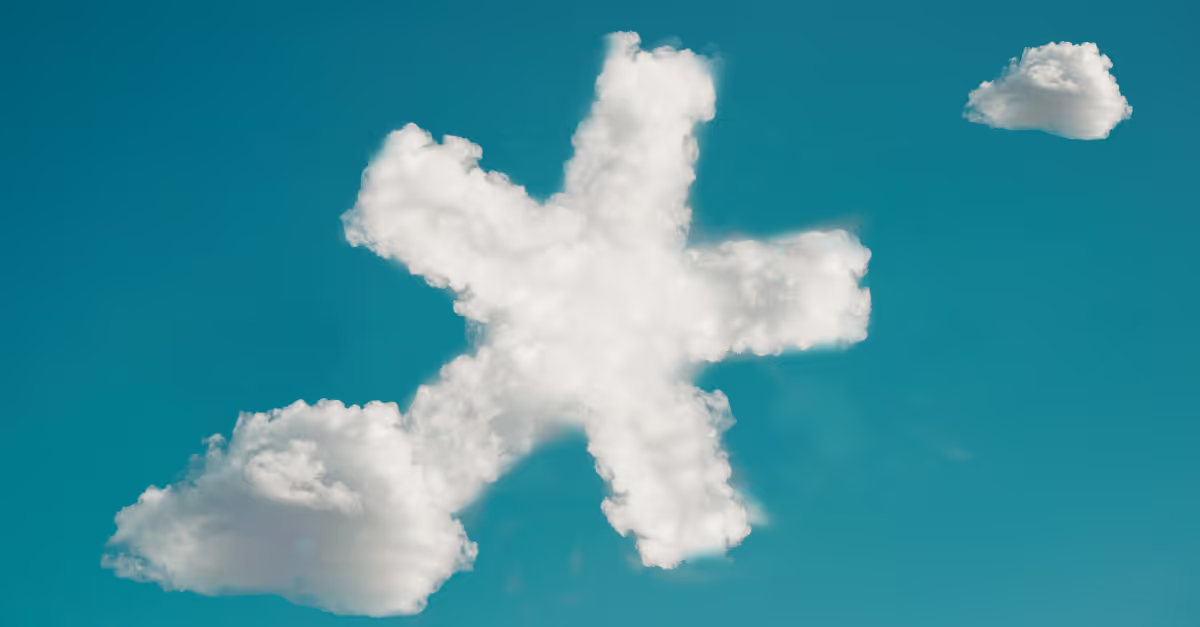